You signed in with another tab or window. Reload to refresh your session.You signed out in another tab or window. Reload to refresh your session.You switched accounts on another tab or window. Reload to refresh your session.Dismiss alert
Hi, thanks for sharing the great work. I get the weight distribution for my own project, and here is my weight distribution:
I wonder what threshold should I set? I am confused......
The text was updated successfully, but these errors were encountered:
Ariel-JUAN
changed the title
How to draw weight distribution?
How to set threshold for pruning?
Nov 19, 2018
Hi, thanks for sharing the great work again. I spent some time reading your source code. I have something confused. I prune the model under the threshold, and then what I need to do is finetune the model. I saw you provide deploy_pruned_model.py,
`
`
It looks like the weights equals==0 are still in model, so the model size won't be small, right?
And tf.matmul(net, weights) to get output step by step. If the model is ResNet this kind of multi-branch, it looks like it doesn't work==
If you have time, please take a look at it and reply me, thanks~
Hi, thanks for sharing the great work. I get the weight distribution for my own project, and here is my weight distribution:
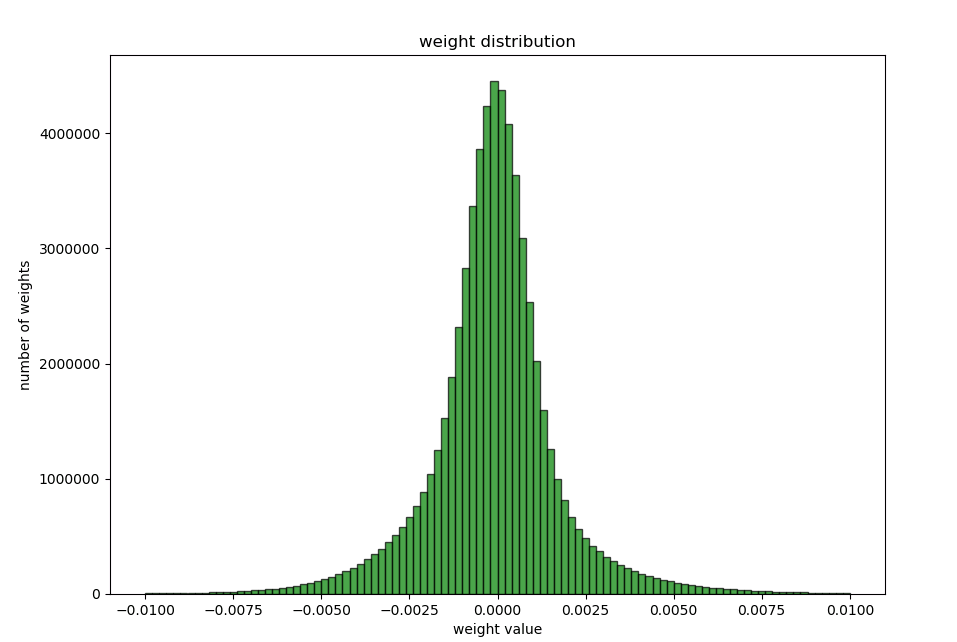
I wonder what threshold should I set? I am confused......
The text was updated successfully, but these errors were encountered: